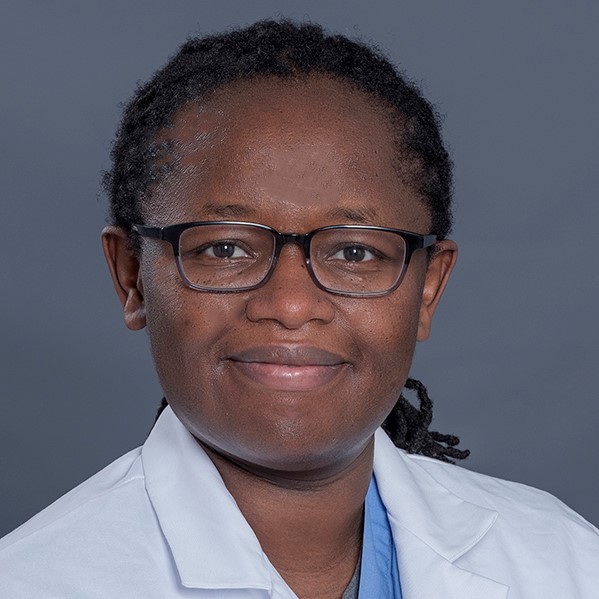
Emory radiologist Judy Gichoya led a team of researchers who determined that artificial intelligence models can detect a patient's race, but they don't know how the machines figure it out.
Can computers figure out your race by looking at your wrist bones or lungs? Yes, according to a study published this month by the prestigious scientific journal, The Lancet Digital Health. That’s not the whole story, though: the bigger issue is researchers don’t know how the machines do it.
The findings come after months of work by a team of experts in radiology and computer science led by Judy W. Gichoya, MD, assistant professor and director of the Healthcare Innovations and Translational Informatics Lab in Emory University School of Medicine’s Department of Radiology and Imaging Sciences. Additional Emory researchers include Hari Trivedi, MD, assistant professor of radiology and imaging sciences, Ananth Bhimireddy, MS, systems software engineer and computer science student Zachary Zaiman. The team also includes colleagues from Georgia Tech, MIT, Stanford, Indiana University-Perdue University and Arizona State, plus experts in Canada, Taiwan and Australia.
The team used large-scale medical imaging datasets from both public and private sources, datasets with thousands of chest x-rays, chest CT scans, mammograms, hand x-rays and spinal x-rays from racially diverse patient populations.
They found that standard deep learning models — computer models developed to help speed the task of reading and detecting things like fractures in bones and pneumonia in lungs — could predict with startling accuracy the self-reported race of a patient from a radiologic image, despite the image having no patient information associated with it.
“The real danger is the potential for reinforcing race-based disparities in the quality of care patients receive,” says Gichoya. “In radiology, when we are looking at x-rays and MRIs to determine the presence or absence of disease or injury, a patient’s race is not relevant to that task. We call that being race agnostic: we don’t know, and don’t need to know someone’s race to detect a cancerous tumor in a CT or a bone fracture in an x-ray.”
The immediate question was whether the models, also known as artificial intelligence (AI), were determining race based on what researchers call surrogate covariables. Breast density, for example, tends to be higher in African American women than in white women, and research shows Black patients tend to have higher bone mineral density than white patients, so were the machines reading breast tissue density or bone minerality as proxies for race? The researchers tested this theory by suppressing the availability of such information to the AI processor and it still predicted patient race with alarming accuracy: more than 90 percent accurate.
Even more surprising, the AI models could determine race more accurately than complex statistical analyses developed specifically to predict race based on age, sex, gender, body mass and even disease diagnoses.
The AI models worked just as well on x-rays, mammograms and CT scans and were effective no matter which body part was imaged. Finally, the deep learning models still correctly predicted self-reported race when images were deliberately degraded to ensure the quality and age of the imaging equipment wasn’t signaling socioeconomic status, which in turn could correlate with race. Fuzzy images, high resolution images downgrades to low resolution, and scans clipped to remove certain features did not significantly affect the AI models’ ability to predict a patient’s race.
So what?
While unconscious bias for radiologic interpretation is not well understood, unconscious biases about racial groups have resulted in well-documented differences in the quality and kind of health care people receive in other specialties due to race.
Finding ways to lighten increasing radiology workloads is a major reason why radiologists are turning to deep machine learning or AI: can certain diagnostic tasks be automated so radiologists can spend more time on complex cases? That’s the hope — and the danger.
“We don’t know how the machines are detecting race so we can’t develop an easy solution,” says Gichoya. “Just as with human behavior, there’s not a simple solution to fixing bias in machine learning. The worst thing you can do is try to simplify a complex problem.”
Publication by The Lancet Digital Health, which is peer-reviewed, is powerful validation of the research itself and of its implications, according to the researchers.
The real fear, Gichoya says, is that all AI model deployments in medical imaging are at great risk for causing great harm.
“If an AI model starts to rely on its ability to detect racial identity to make medical decisions, but in doing so produces race-specific errors, clinical radiologists will not be able to tell, thereby possibly leading to errors in health-care decision processes. That will worsen the already significant health disparities we now see in our health care system,” explains Gichoya.
And because of that danger, the team already is working on a second study. They will not stop at detecting bias, Gichoya says. “This ability to read race could be used to develop models that actually mitigate bias, once we understand it. We can harness it for good.”