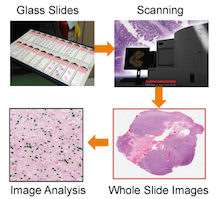
Emory computer scientist Fusheng Wang, PhD has been awarded a five-year, $446,000 grant from the National Science Foundation to support software development in the field of "spatial big data." The Faculty Early Career Development (CAREER) award is the NSF's most prestigious award in support of junior faculty who exemplify the role of teacher-scholars.
Wang is an assistant professor of biomedical informatics at Emory University School of Medicine. Before coming to Emory in 2009, he was a research scientist at Siemens Corporate Research.
Wang's project aims to bridge the fields of spatial data management and high performance computing, and to develop open source software for use by investigators in multiple disciplines. These tools could help attack problems ranging from cancer diagnosis to wildfires and traffic congestion.
Software developed by Wang and his team could analyze massive spatial data coming from diverse sources, such as high resolution microscopic images derived from pathology samples, satellite imagery of the earth, social media, or "crowd-sourced" data from smart phones or other GPS receiving devices.
"The challenges for big data come not only from the volume but also the complexity, such as the multi-dimensional nature of data," Wang says. "With fast increasing processing power of computers at decreasing price, we envision a future in which processing big spatial data is as fast as how we are processing ordinary data today."
In the realm of education, the grant will support new undergraduate and graduate courses on big data management, symposia and science projects for K-12 students.
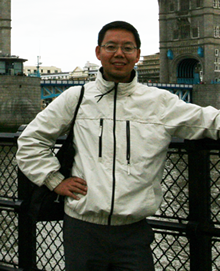
Fusheng Wang
One of the purposes of Wang's project is to develop high performance distributed computing tools, allowing multiple computers to contribute to spatial big data problem solving in commodity clusters or cloud environments. He is planning to use a popular framework called MapReduce (or Hadoop, its open source implementation) for efficiently handling massive spatial datasets.
"The MapReduce based computing model provides a highly scalable, reliable, elastic and cost effective framework for storing and processing massive data on a cluster or cloud environment," Wang says. "However, spatial queries and analytics are intrinsically complex and difficult to fit into the model due to its multi-dimensional nature, so there are major gaps that we plan to address."
Wang and his colleagues also plan to adapt hybrid CPU-GPU systems (computer systems with dedicated graphics processing units) for MapReduce and spatial data processing.